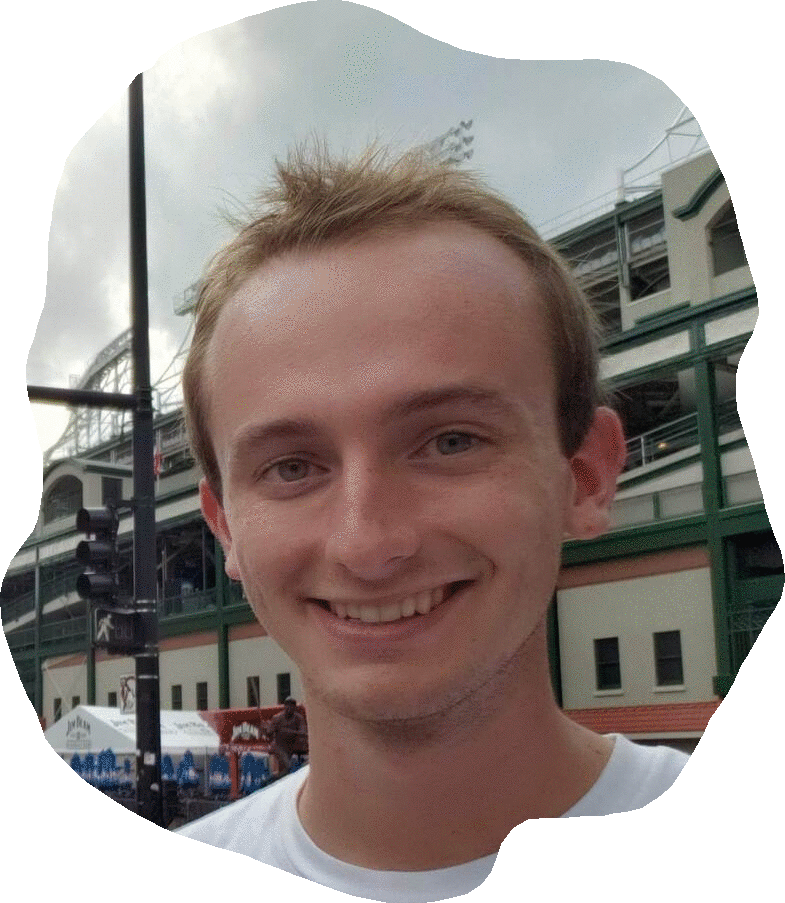
I'm a second-year computer science PhD student at DIRO at the Université de Montréal, studying machine learning and NLP. I am advised by Bang Liu, and I'm currently working on augmenting large language models (LLMs) to better interact with procedural knowledge. Here's my resume (and the academic version).
Previously I was a speech scientist at Cobalt Speech & Language, a company that designs custom speech recognition, text-to-speech, and dialogue models. While at Cobalt I worked on some neat projects, including language modeling for recognizing air traffic control speech and creating an online training system for ASR models.
I graduated from BYU with a BS in Applied and Computational Mathematics (ACME) with an emphasis in linguistics and a minor in computer science. ACME's rigorous curriculum includes graduate-level courses in algorithms, analysis, optimization, statistics, data science, optimal control, and machine learning.
During my undergrad I interned with Cobalt Speech, as well as Emergent Trading, an automated trading firm that made the news for reporting a problem in a Eurodollar exchange rule that unfairly favored larger competitors. (I developed the analysis tools that were used to track the issue down and determine how our opponent was taking advantage of the rule.)
contact (he/him)
Around the web I'm known by the username kylrth
. I prefer to be contacted through
the Matrix protocol (@kyle:kylrth.com
). (If you'd
like an account on my Matrix server, follow the instructions here.) My GPG public key is here.
Matrix  /  email  /  GitHub  /  LinkedIn  /  phone  /  WhatsApp  /  Signal  /  Session
research
Here's my academic CV.
I'm currently developing methods to augment large language models to more effectively produce coherent procedures when the knowledge comes from retrieved documents. This is a Mitacs collaboration with Thales Canada.
other projects
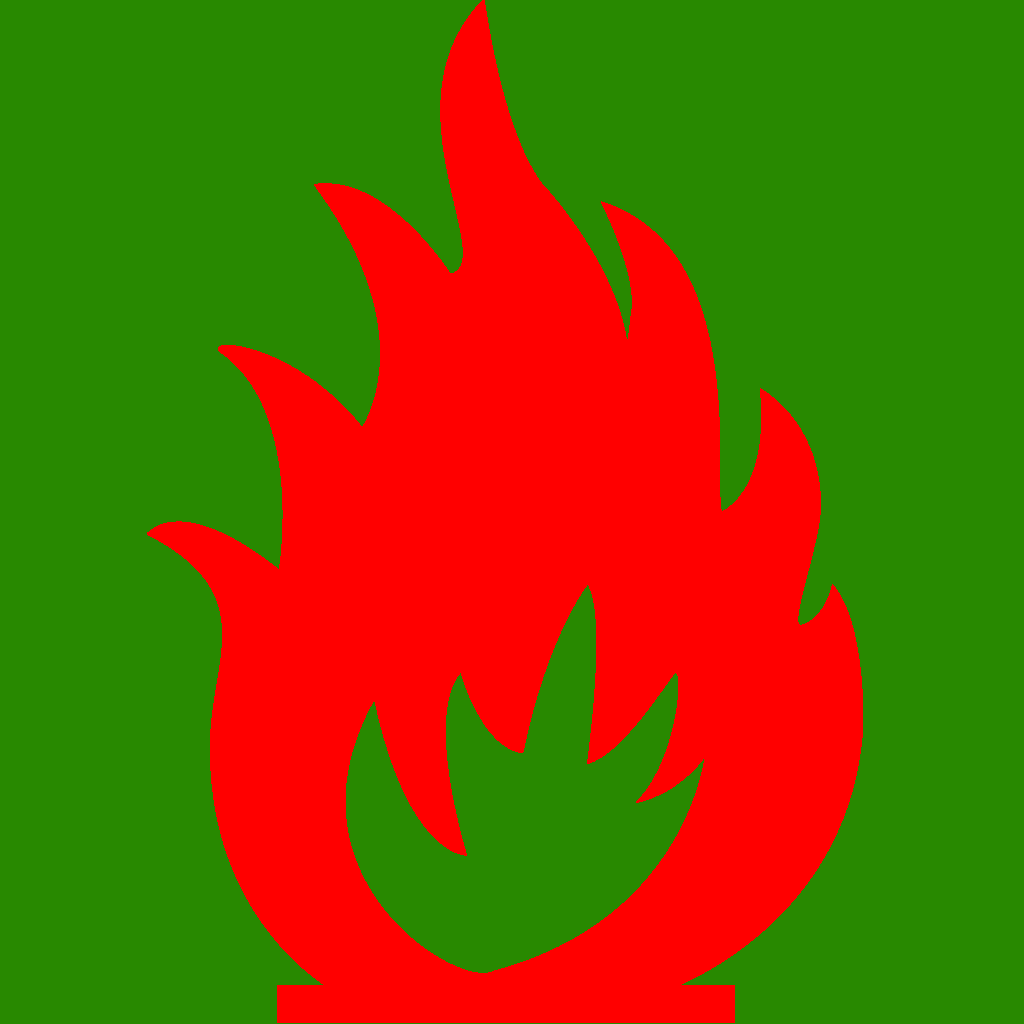
