This was mentioned in the paper on Google’s Multilingual Neural Machine Translation System. It’s regarded as the original paper to use the word-piece model, which is the focus of my notes here.
the WordPieceModel
Here’s the WordPieceModel algorithm:
func WordPieceModel(D, chars, n, threshold) -> inventory:
# D: training data
# n: user-specified number of word units (often 200k)
# chars: unicode characters used in the language (e.g. Kanji, Hiragana, Katakana, ASCII for Japanese)
# threshold: stopping criterion for likelihood increase
# inventory: the set of word units created by the model
inventory := chars
likelihood := +INF
while len(inventory) < n && likelihood >= threshold:
lm := LM(inventory, D)
inventory += argmax_{combined word unit}(lm.likelihood_{inventory + combined word unit}(D))
likelihood = lm.likelihood_{inventory}(D)
return inventory
The algorithm can be optimized by
- testing only word pairs that exist in the training data
- testing only pairs with a significant chance of being the best
- combining several clustering steps into a single iteration (possible for groups of pairs that don’t affect each other)
- only modify the LM counts for affected entries
After these optimizations, building a 200k word piece inventory can take a few hours on a single machine.
Dealing with spaces
They also do something important to make sure the ASR output text has spaces formatted reasonably. It’s best explained in the following image from the paper:
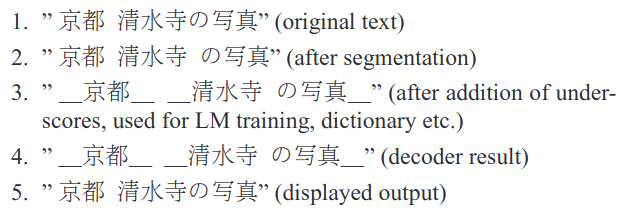
LM
They use entropy-pruned 3- to 5-grams with Katz back-off after removing unwanted symbols etc. as much as possible similar to what is described in a previous voice search paper from Google.
pronunciation dictionary
They used a hodge-podge of various techniques to generate the pronunciation dictionaries.
- IME data
- extractions of readings from the web
- a transliterator for loan words
- rule-based approaches
- reviewing by hand the most important groups of pronunciations